Who: Jiacheng Liang
Abstract: To mitigate the misuse of large language models (LLMs), such as disinformation, automated phishing, and academic cheating, there is a pressing need for the capability of identifying LLM-generated texts. Watermarking emerges as one promising solution: it plants statistical signals into LLMs' generative processes and subsequently verifies whether LLMs produce given texts. Various watermarking methods ("watermarkers") have been proposed; yet, due to the lack of unified evaluation platforms, many critical questions remain under-explored: i) What are the strengths/limitations of various watermarkers, especially their attack robustness? ii) How do various design choices impact their robustness? iii) How to optimally operate watermarkers in adversarial environments?
To fill this gap, I systematize existing LLM watermarkers and watermark removal attacks, mapping out their design spaces. I then develop WaterPark, a unified platform that integrates 10 state-of-the-art watermarkers and 12 representative attacks. More importantly, leveraging WaterPark, I conduct a comprehensive assessment of existing watermarkers, unveiling the impact of various design choices on their attack robustness. For instance, a watermarker's resilience to increasingly intensive attacks hinges on its context dependency. I further explore the best practices to operate watermarkers in adversarial environments. For instance, using a generic detector alongside a watermark-specific detector improves the security of vulnerable watermarkers. I believe my study sheds light on current LLM watermarking techniques while WaterPark serves as a valuable testbed to facilitate future research.
ZOOM: https://stonybrook.zoom.us/j/98907127969?pwd=a7TeLBznnbqNTsWr6kSLXZQNHEfADu.1
Meeting ID: 989 0712 7969
Passcode: 297515
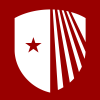