Towards Addressing Challenges in Developing Resources for Evaluating Semantic Understanding
NLP models are increasingly deployed in critical applications. However, recent studies have shown that their performance can often be attributed to merely exploiting surface-level patterns in the text. Therefore it is essential to develop tasks and datasets to carefully access models on the nuanced aspects of the semantic understanding of interest. Through three research projects, my thesis will highlight and address some of the challenges in creating semantic understanding-based resources for diverse text domains.
First, I focus on the semantics of implicit participant states in simple narratives. We introduce PASTA, a crowdsourced dataset that captures knowledge needed to infer implicit participant states and understand the impact of changes to these states on the narrative. To capture these aspects of state-based reasoning, we developed a data annotation pipeline where human annotators performed multi-step commonsense reasoning tasks. Based on the annotation, we propose three state-based reasoning tasks. Our data annotation pipeline produces contrastive task instances, preventing common unwanted annotation artifacts. Benchmarking results on the task shows significant room for improvement, especially in narratives requiring diverse types of knowledge for comprehension. My second project focuses on the semantics of Network File System specification texts (NFS-RFC). We introduce SpecNFS, a resource designed to evaluate models' understanding of programmatic semantics needed to express constraints and their logical relations within NFS-RFC texts. We design an intermediate system-agnostic representation, SpecIR, a semantic dependency parse structure that captures these semantics. We propose semantic role and dependency link labelling tasks over the semantic parse structures. Following an iterative data annotation protocol, we curate a dataset SpecNFS, containing NFS specifications and their SpecIR representation, which is used to benchmark existing models on the tasks.
Finally, my proposed work aims to evaluate theory of mind semantics and reasoning over variables in multi-party conversations, with information asymmetry amongst participants. I am specifically interested in conversations with variables with changing values across the conversation. We introduce a conversation comprehension task requiring models to simultaneously track and reason over multiple dynamic variables with each participant having a different mental state of the world. To address the challenge of curating such highly specific and constrained conversation datasets, I plan to leverage LLMs in a multi-step data generation pipeline. Through this resource we want to thoroughly evaluate models on the complexities of real world conversations.
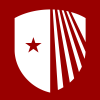