Abstract:
Human priors play a crucial role in visual recognition systems, offering numerous advantages. They help to reduce reliance on extensive training datasets, provide users more control over the systems' output, and enhance our understanding of the systems' output. In this thesis, I focus on designing machine learning methods that enable human priors in the training phase to improve the model performance, and in the inference phase to improve the quality of individual outputs. The methods concentrate on facilitating priors which are convenient for humans to obtain and to provide.
Firstly, I propose a novel approach to leverage human prior knowledge on the visual similarity relationships between categories for synthesizing data in few-shot learning problems. Specifically, I propose RelationVAE, a generative model trained by optimizing the data likelihood on a graphical model which encodes class relationships. RelationVAE is used to synthesize training data for few-shot categories. As a secondary focus, I introduce a segmentation technique that enables human users to supply prior knowledge about the segmentor's sensitivity for each single input during the inference time. The proposed method incorporates a numerical parameter into the segmentor, enabling it to react accordingly to varying levels of sensitivity. In my third contribution, I propose a methodology tailored for the few-shot fine-grained counting task. This method enables human users to input prior knowledge about the granularity level of the target category, ranging continuously from coarse to fine. This prior knowledge is fed into the proposed model through a parameter which modulates the category granularity of the result. Finally, my future plan involves developing a counting model that automatically identifies and counts repeating objects at various granularity levels without the need for human exemplar input. Additionally, it will enable interaction, allowing users to choose counting results for their objects of interest at their preferred level of granularity.
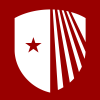