Abstract:
People shift their visual attention to gather and prioritize information from their surroundings, aiding in effective adaption and navigation through complex environments. Decoding attentional processes entails exploring the what, where, and when of attention guidance, that involves understanding the stimuli that attract attention (what), the spatial distribution of attention (where), and the temporal dynamics of attention shifts (when). Addressing these questions offers valuable insights into attentional mechanisms, crucial for various applications, spanning from designing effective user interfaces to aiding medical diagnoses. However, previous works that predict visual attention have not fully explored the underlying factors addressing these three questions.
In this thesis, we study the underlying factors that influence attention guidance across diverse image types, spanning natural images, graphic design documents, and whole slide images (WSIs) of cancer tissues, while also predicting visual attention based on these factors. First, we propose a novel approach to quantify object recognition uncertainty (what), which we find has a greater role than bottom-up saliency in guiding overt attention during natural image viewing. Second, we extend our investigation to include graphic design documents (\eg~webpages, comics, posters, mobile UIs, etc.), which differ from natural images in their composition, designed to convey specific messages or elicit desired viewer response. We present a unified and interpretabledeeplearning model for predicting static and dynamic visual attention behavior (addressing the where and when questions) during the free viewing of such documents by integrating saliency of document components and layout information (addressing what) to enhance attention prediction performance. Third, in the domain of digital histopathology, we investigate pathologists' attention during their examination of giga-pixel WSIs of prostate cancer, which is useful in developing computer-assisted training and clinical decision support systems. We find that pathologist attention is influenced by diverse factors such as viewing magnification, slide staining, nature of the task, expertise of the pathologist, etc. Using our web-based digital microscope, we collect the largest known dataset of pathologist attention, which allows us to traindeeplearning models for predicting pathologist attention as well as predicting their expertise level based on their spatio-temporal attention patterns.
While our study addresses the where (spatial distribution of attention in WSI) and when (timeline of viewing WSI regions) of pathologist attention, the question of what pathologists look at, in terms of interpretable visual content (\eg~tumor subtype, pathomic features, etc.) during their WSI reading remains unexplored. To conclude this thesis, we propose to investigate this direction as our future study.
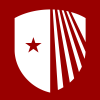