Abstract:
Much of natural language is generated by humans who take on many different states and traits over their lifetime. However, little research has been done with respect to analyzing language in the temporal dimension. While there is a small collection of works focused around human-level natural language processing (NLP), many of these largely ignore problems related to tracking change in states or language use itself over time. Instead they opt to perform analysis in a cross-sectional manner, by modeling language from one group and applying the learned model to an out-of-sample population. These previous works have set the foundation on ways to best analyze language unique to an individual or community, but they simply do not do much in the way of tracking changes cross-time.
The push towards making natural language processing account for the person behind the language has been moderately successful. Techniques that allow for one's language to be understood in context of their age and gender (Zamani, 2018), applications for automatic assessment of mental health (Matero et al. 2019; De et al., 2013; Lynn et al., 2018), and predicting a person's sentiment or stance towards or about an entity (Matero et al., 2021; Lynn et al., 2019) have all become popular over the past decade. Many of these works have been made possible by the abundance of publicly available social media data or through recruitment where someone could willingly release their data and possibly perform some baseline survey or questionnaire (Schwartz et al., 2013; Eichstaedt et al., 2018, De et al. 2016;). However, most of these works ignore the temporal dimension and those that do consider it are not modeling time as a sequence but rather look to make a singular forecast into the future based on all currently available data (Jose et al., 2022).
This thesis covers work developing new longitudinal natural language processing neural network architectures, by adapting current sequential models, as well as the introduction of temporally aware language-based tasks.The proposed models and tasks have been developed over various sub-domains of social media, such as Twitter and Facebook, and have shown great utility in their goal for modeling not only changing states of individuals but also behavior of communities at large (e.g. U.S. Counties).
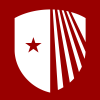