Abstract:
Over the past decade, Cardiovascular Magnetic Resonance (CMR) imaging has played an essential role in diagnosing heart diseases, evaluating disease progression, and guiding treatment strategies. Recently, substantial advancements in deep learning technology have significantly enhanced its performance in supporting biomedical research and clinical decision-making, particularly in areas such as image reconstruction, recognition, segmentation, and synthesis. Despite these advancements, information acquisition from CMR still faces several limitations, such as long data acquisition time, poor image reconstruction quality, and complex types of CMR images. To deal with these challenges, we proposed some general approaches to improve the accuracy and efficiency of CMR information acquisition.
In this report, we present three projects aimed at efficiently obtaining CMR information from raw data or other types of CMR images to assist physicians in the diagnosis of cardiovascular disease. In the first project, we developed a novel CNN-based deep learning framework for ventricular volumetric measurements directly from real-time CMR raw data without image reconstruction. This work demonstrated that the framework gave better volumetric measurements than those with conventional methods based on image reconstruction and segmentation. In addition, the presented 'image-less' CNN model provided a gain in imaging acceleration for volumetric measurements over the conventional image-based deep learning models. In the Second project, we developed a multiscale feature learning model, SwinUNet, for parametric mapping of T1 and T2 relaxation times with CMR. This model gave an optimal solution to accelerating CMR parametric mapping, making it possible to scan more heart patients for myocardial tissue characterization in the daily practice of clinical cardiology. In the third project, we proposed a multiscale perception model for learning the nonlinear relationship between flow velocity and MRI magnitude in dynamic images collected without velocity encoding. The results showed that deep-learning flow imaging was feasible without velocity encoding. By collecting fewer images, a gain in temporal resolution was achieved for through-plane flow measurements in the major blood vessels.
We conclude this report by discussing the main challenges in synthesizing contrast-enhanced CMR images from CMR cine image time series, our plans to address these issues, and future directions.
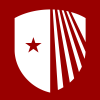