Dates
Thursday, December 08, 2022 - 10:00am to Thursday, December 08, 2022 - 12:00pm
Location
NCS 120
Event Description
Abstract:
The prediction of human attention will enable human-computer interaction systems to better anticipate a person's needs and intents. Attention control takes bottom-up and top-down forms that are typically studied in separate free-viewing and visual search literatures, respectively. However, most models have mainly focused on predicting free-viewing behavior using {\it saliency maps}, but these predictions do not generalize to goal-directed behavior, such as when a person searches for a visual target object (visual search). In this thesis we explore ways to build models that predict human attention control in the form of {\it fixation scanpath} for both the goal-directed visual search and \enquote{taskless} free viewing. Meanwhile, we require high interpretability of the models which serve as a tool for understanding human gaze behavior.
At the core of the scanpath prediction problem is a question of how to represent the knowledge that is dynamically updated by the visual information humans perceive at every fixation of a scanpath. To address this problem, we first present a straightforward method of representing dynamic knowledge in the human brain during visual search by integrating the visual information acquired at each fixation via Cumulative Foveated Image (CFI). Second, to improve the interpretability of the model, we propose to represent the viewer's internal belief states as dynamic contextual belief maps of object locations, which we call Dynamic Contextual Beliefs (DCBs). We also propose the first inverse reinforcement learning (IRL) model to learn the internal reward function and policy used by humans during visual search. The reward maps recovered by the IRL model reveal distinctive target-dependent patterns of object prioritization, which we interpret as a learned object context. Third, to avoid applying CNNs pretrained on full-resolution images on blurred images as in CFI and DCBs, which makes the representation unstable, we propose Foveated Feature Maps (FFMs). FFMs leverage the inherent hierarchical architecture in modern CNNs and combine the hierarchical feature maps in a manner that is contingent upon the human fixation locations, approximating the information available from a foveated retina. Finally, to address the lack of temporal and spatial information in the representations like CFI, DCBs and FFMs, we propose Foveated Working Memory (FWM) integrated into a transformer-based model, Human Attention Transformer (HAT) that unifies both bottom-up and top-down forms of attention control by predicting the scanpath (a sequence of fixations) made during both visual search and free viewing. Critical to HAT's effectiveness and scope is a novel transformer-based design and a simplified foveated retina that collectively work to create a form of spatiotemporal-aware, dynamically-updating, foveated visual working memory.
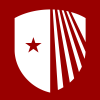
Event Title
Ph.D. Proposal Defense: Zhibo Yang, 'Learning Representations for Human Attention Control Prediction'