Abstract
Bayesian models are models that use probability distributions to quantify the uncertainty in parameter estimation and model predictions (i.e., movement time MT). In this research, we apply Bayesian modeling to model two basic interaction actions: pointing and steering. The former is about acquiring a target with a mouse pointer, and the latter is about tracing through a tunnel using a mouse pointer.
We first investigate how to model the distribution of movement time (MT) for steering actions. We propose a quadratic variance model which reveals that the variance of MT is quadratically related to ID with the linear coefficient being 0. Empirical evaluation on a new and a previously collected dataset shows that the quadratic variance model accounts for between 78% and 97% of the variance of observed MT variances; it outperforms other model candidates such as linear and constant models; adding the linear coefficient leads to no improvement on the model fitness. The variance model enables predicting the distribution of MT given ID: we can use the variance model to predict the variance parameter and Steering law to predict the mean parameter of a distribution. We have evaluated six types of distributions for predicting the distribution of MT. Qur research advances the MT prediction of steering tasks from a point estimate to variance and distribution estimates, which provides a more complete understanding of steering behavior and quantifies the uncertainty of MT prediction.
Second, we extend Fitts' law, a classic pointing model, to be a Bayesian hierarchical pointing model and compare it with the typical pooled pointing models (i.e., treating all observations as the same pool), and the individual pointing models (i.e., building an individual model for each user separately). The Bayesian hierarchical pointing models outperform pooled and individual pointing models in predicting the distribution and the mean of pointing movement time, especially when the training data are sparse. Our investigation also shows that both noninformative and weakly informative priors are adequate for modeling pointing actions, although the weakly informative prior performs slightly better than the noninformative prior when the training data size is small. Overall, we conclude that the expected advantages of Bayesian hierarchical models hold for the pointing tasks. Bayesian hierarchical modeling should be adopted a more principled and effective approach of building pointing models than the current common practices in HCI which use pooled or individual models.
Third, we are applying Bayesian hierarchical pointing models to understand the motor control ability of patients with Parkinson's Disease and healthy older adults (age >= 65). Since patients with Parkinson's Disease have motor impairments that would affect their motor control ability and movement time in interaction, modeling their motor behavior would help detect early signs of this disease.
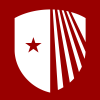