Abstract:
Machine learning has achieved remarkable success in data-intensive applications, yet it still encounters challenges when data is insufficient. To tackle this issue, few-shot learning (FSL) has been proposed. FSL aims to develop methods that can rapidly generalize to new tasks with minimal labeled samples. It is particularly beneficial in scenarios where acquiring labeled data is difficult or impractical.
In this thesis, we explore the use of synthetic data generation techniques to enhance FSL. To achieve this, we employ generative models to capture the data distribution within a continuous latent space, which allows the sampling of new data to facilitate learning in data-scarce scenarios. We demonstrate the capability of this framework to synthesize diverse and reliable data that can enhance FSL across various tasks, including few-shot image classification, fine-grained few-shot classification, few-shot object detection, and class-agnostic object counting. Specifically, for few-shotimage classification, we propose a method to generate reliable data via sample selection. For fine-grained few-shot classification, we propose sampling diverse data representing the distribution of intra-class variance.For few-shot object detection, we focus on how to generate data with increased crop-related diversity. For class-agnostic object counting, we present a method for generating reliable data that can serve as object templates. Finally, to conclude this thesis, we investigate a more general question: under what conditions do generative models produce high-quality samples? To address this, we introduce a method for quality assessment based on latent space analysis, ensuring a more reliable use of generated samples beyond FSL scenarios.
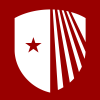