Who: Sayontan Ghosh
Abstract: NLP models are increasingly deployed in critical applications, which necessitates their
systematic evaluation on nuanced aspects of semantic understanding. My thesis highlights
challenges in creating semantic understanding-based resources for diverse text domains
through three research projects. First, I focus on the semantics of implicit participant states
in simple narratives. We introduce PASTA, a dataset that captures inferential and
counterfactual knowledge about these states in context to the narratives. We capture these
aspects of state-based reasoning through a data annotation pipeline where human annotators
perform multi-step commonsense reasoning tasks and introduce three state-based reasoning
tasks. Our data annotation pipeline produces contrastive task instances, preventing common
unwanted annotation artifacts. Benchmarking results reveal significant gaps, especially in
narratives requiring diverse knowledge types. My second project focuses on the semantics of
Network File System specification texts (NFS-RFC). We introduce SpecNFS, a resource
designed to evaluate models' understanding of programmatic constructs needed to express
logical constraints in NFS-RFC texts. We design an intermediate system-agnostic
representation, SpecIR that captures these semantics, and propose semantic role and
dependency link labeling tasks over them. Following an iterative data annotation protocol,
we curate the dataset containing NFS specifications and their SpecIR representation, and
benchmark existing models on the tasks. Finally, my third project focuses on evaluating
dynamic variable state and theory of mind understanding in multiparty conversations. We
present DIAMOND, a conversation comprehension dataset that evaluates these abilities in
models. To curate such highly constrained dataset, we design a multi-step LLM-based data
generation pipeline. Our dataset test critical capabilities, including dynamic information
tracking, long-term dependency resolution, and distractor robustness. Model benchmarking
results shows significant performance gaps in state-of-the-art LLMs, particularly in handling
participant-specific perspectives and distractor-rich contexts.
Zoom link: https://stonybrook.zoom.us/j/92328285452?pwd=WfkwRAZnJKpqAv7lmd4NfBKTuAifBT.1
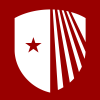