Abstract:
Human action recognition, anticipation, and retrieval are important problems in computer vision with applications in many domains. We can train deep models for these tasks with supervised learning, but the generalization ability of these models depends on the amount of annotated training data. Annotating human actions in videos, however, is a laborious and ambiguous process, so we do not generally have a lot of training data and the trained models have poor generalization ability. In this dissertation, to increase the knowledge of the models trained with the limited amount of training data, I propose to use knowledge distillation to distill complementary knowledge to the models. Specifically, I demonstrate the benefits of knowledge distillation in four different tasks. First, I introduce a method to improve early recognition of human actions with knowledge distillation from a network trained with longer video observation. Second, I propose to use a look-ahead knowledge distillation framework by using the action recognition network on future action segments to transfer the knowledge to the action anticipation network. Third, I improve the video-based action anticipation network by utilizing knowledge from large language models. Finally, I demonstrate that knowledge distillation can be applied across modalities by introducing a novel loss function for the tasks of text-to-video and video-to-text retrieval.
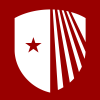