Who: Mahnaz Koupaee
Abstract: Scenarios can be viewed as a collection of events, which alter the world in ways big and small. Modeling events can give us an understanding of how these scenarios unfold and how they can be changed. Real-world scenarios are often complex and immensely diverse. Event language models, which can be trained in a self-supervised manner over a large collection of text sequences, provide a scalable means to acquire the necessary knowledge and to effectively reason about scenarios. However, such event language modeling, when trained only on observed discourse sequences, has serious limitations: (1) it is constrained to follow the discourse order while there are many notions of ordering, (2) it fails to provide mechanisms for guided generation towards areas of interest, and (3) it fails to provide the reasoning for why certain events are likely to occur. The discourse sequences while suggestive of event relations are not reflective of their true logical connections. My thesis aims to retain the scalability aspects of training over discourse sequences, but (i) adds data augmentation to improve generalizability to other domains, (ii) uses structured exploration via entity roles towards controllability for a systematic exploration of scenarios, and (iii) employs semantic relations guided reasoning to improve explainability for event reasoning.
Zoom link: https://stonybrook.zoom.us/j/95718089136?pwd=43SWBB4xpe22hCCyvnoL5pmn1b0uI0.1
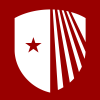